Data is the natural byproduct of transactions, observations, and interactions. At an estimated 2.5 quintillion bytes per day,1 the volume of data generated is on an order of magnitude which makes the national debt look minuscule.
Companies glean value by using data for a variety of purposes, depending on industry and maturity. Manufacturers typically use data for quality control (e.g., Six Sigma) and optimization. Companies across industries rely on data for reports and dashboards to assess various metrics, from key performance indicator (KPI) summaries to complex market segmentation assessment trends.
Those applications of data help companies monitor and measure their current revenue streams. Yet data productization (also referred to as monetization) has the potential to enhance revenues of existing products or open entirely new revenue streams. (See sidebar.)
But what is data “productizing,” and what does the process entail? In essence, Data Productization is a unique mix of Product Management and Data Analytics. And it involves the same business principles that are employed in the development of any new product applications. The difference is that the data and the insights provided are raw material.
As with other products, success in the market depends on product fundamentals such as relevance to consumers, ease of use, uniqueness (i.e., differentiation), etc. However, working with data entails a host of unique considerations. Below, we will explore and review the key components needed to bring your data product ambitions to life.
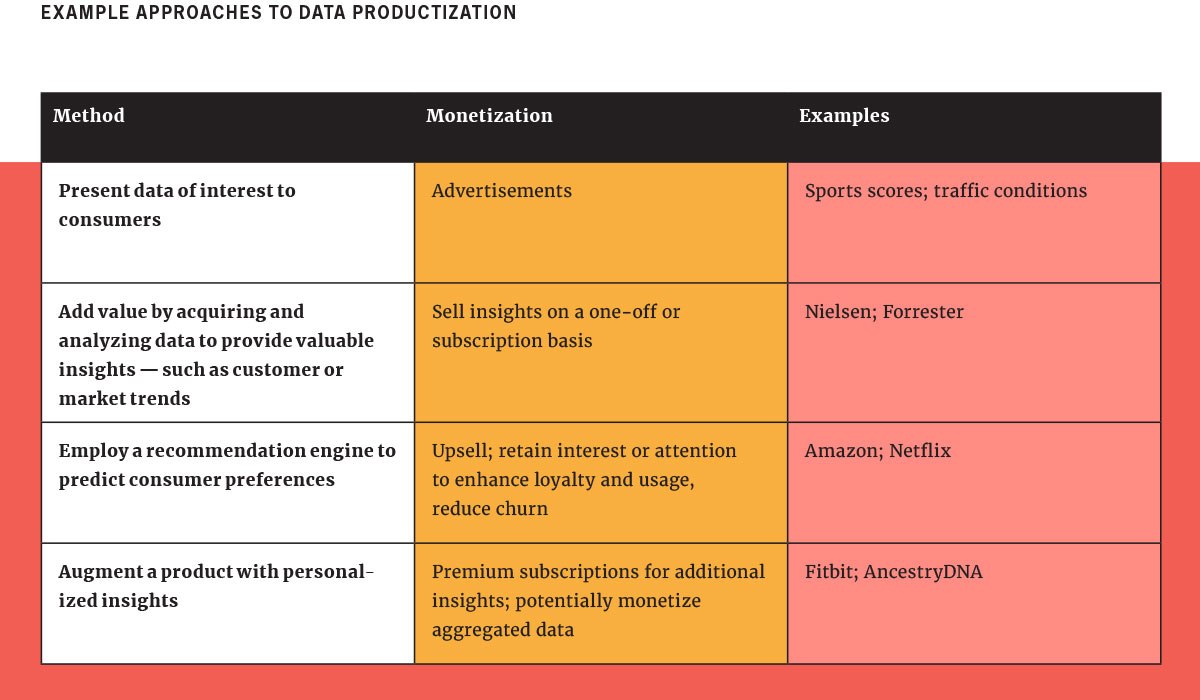
Method
- Present data of interest to consumers
- Add value by acquiring and analyzing data to provide valuable insights — such as customer or market trends
- Employ a recommendation engine to predict consumer preferencesAugment a product with personalized insights
Monetization
- Advertisements
- Sell insights on a one-off or subscription basis
- Upsell; retain interest or attention to enhance loyalty and usage, reduce churn
- Premium subscriptions for additional insights; potentially monetize aggregated data
Examples
- Sports scores; traffic conditions
- Nielsen; Forrester
- Amazon; Netflix
FIVE KEYS TO PRODUCTIZING DATA
1.
Data Storage and Access Are Priority No. 1
Decreased storage costs have allowed companies to create vast reservoirs of data. Some may be on-premises, others in the cloud. But now that data collection is no longer constrained by the ability to store data, new challenges have arisen.
In addition to issues related to architectural decisions around configuration, storage, and retrieval, the problem of “wayfinding” to know where your data resides is not uncommon. This problem can be compounded when servers, data marts, or other infrastructure have been added piecemeal over time to meet tactical needs — creating a labyrinth of overlapping repositories. Additionally, the lack of uniform data definitions across business units, or changes to those definitions over time, can make the data lake murky indeed.
Without question, the most challenging aspect of any project using data is the ability to access it. This is why your efforts to productize data must start with three key goals: (1) understanding what data is available and where it resides; (2) ensuring that there are clear and consistent definitions to identify said relevant data; and (3) knowing how to extract it. These essentials constitute your starting point.
2.
Data Governance Defines the Way Your Data ‘Speaks’
From there, rules must be applied to govern the way data is made to “speak” or be used, and these rules are illustrated in the Data Productization Pyramid (see Figure 1). This may seem ancillary to the subject of data productization; however, it is a critical consideration that determines what can be done with the data to which you now have access.
A comprehensive definition of Data Governance is “a system of decision rights and accountabilities for information-related processes, executed according to agreed-upon models which describe who can take what actions with what information, and when, under what circumstance, using what methods.”2
Our “Jabian Information Equation” addresses the entire spectrum of data governance and includes considerations related to data ownership, management, control, access, and compliance. In this article, we will focus on access and utilization, which are key in using data to develop products.
There are several potential considerations around access to and usage of data. Some may be contractual; others may be related to privacy concerns. There may also be internal business rules that govern what group can see particular data sets or reports. Likewise, there may be reasons why certain data sets cannot be combined — for example, the resultant data set would provide insights to which the user should not have access.
Further, defining and enforcing who can access specific data, and how it may be used, are critical when it comes to complying with various data privacy regimes in which a company operates. No one should waste a single cycle of data productization efforts until the rights of data ownership and usage permissions are clearly understood.
3.
Who Does What? How to Define Key Data Roles
Working with data requires an ever-evolving group of individuals and job titles. Data architects, engineers, and developers build and maintain the infrastructure for data acquisition, generation, storage, retrieval, archiving, and disposition. User researchers help you understand your customers’ goals and how they interact with your product, while user experience designers make it consumable. Neglecting these two positions — user researchers and experience designers — might improve speed to market initially but will likely decrease customer experience, loyalty, and, inevitably, cause your data product to fall flat. Meanwhile, data analysts and data scientists turn the data into insights for consumers.
Lastly, the Product Management team directs and coordinates activities — such as problem and solution discovery, solution planning, prioritization, etc. For data productization projects, this role requires both someone with the business acumen to recognize, evaluate, and drive new product opportunities and someone who is well-versed in the possibilities data and insights can provide. The individual need not be a data scientist, but they must be able to articulate the product goals and requirements to those who work the magic of programming artificial intelligence (AI) and machine learning (ML) algorithms.
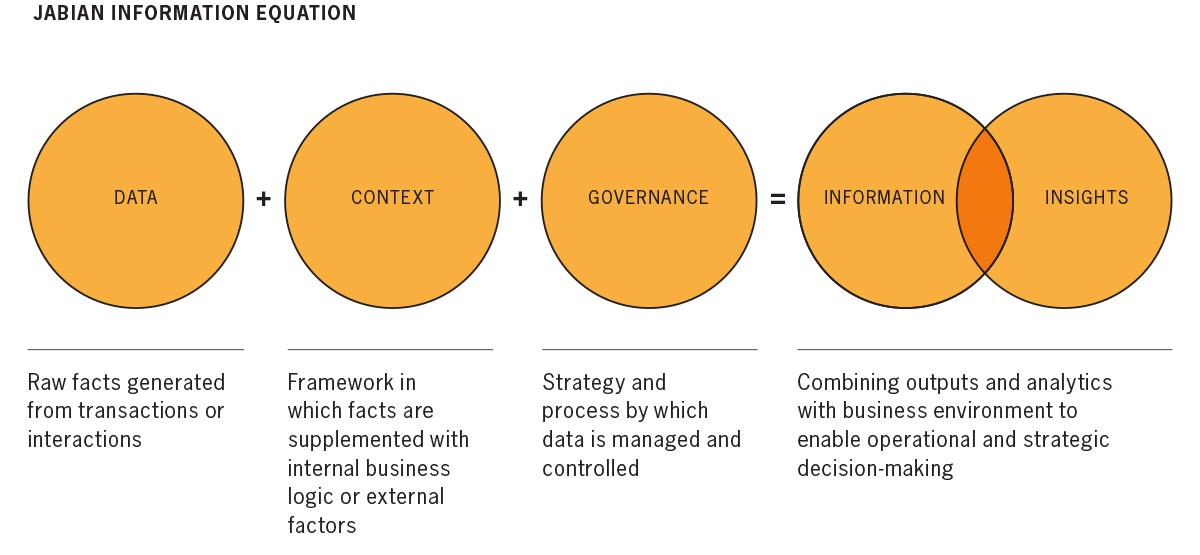
JABIAN INFORMATION EQUATION
DATA +
Raw facts generated from transactions or interactions
+ CONTEXT
Framework in which facts are supplemented with internal business logic or external factors
+ GOVERNANCE
Strategy and process by which data is man- aged and controlled
= INFORMATION INSIGHTS
Combining outputs and analytics with business environment to enable operational and strategic decision-making
4.
Integration and Data Sharing Generates New Product Ideas
Integrating and sharing data throughout your organization will help generate new ideas and maximize the reach of your data productization efforts. Here are some ways to achieve harmony among your organization’s key actors:
- Self-service. Whether it’s via interactive dashboards, reporting, or logging, provide ways for your audience to engage. Automation and self-service capabilities drive efficiency and idea creation.
- Provide an easily accessible, centralized tool to locate and access raw data, definitions, and common tables throughout your organization (e.g., Infosys Analytics Workbench). Consistency and ease of locating data are key for efficiency and data literacy.
- Maintain a catalog to keep track of what systems are being used within your organization (e.g., Collibra). End-users can access this catalog to locate data tools, and data governors can reference it to understand how users are applying data and if new systems are being adopted for unknown reasons (i.e., user research).
- Provide a central tool that creates an immediate feedback loop — questions, requests for improvement, and data support — for continuous improvement.
- Enhance data literacy with training and repetitive communication to ensure the above measures are adopted and are successful. Why go through all of these efforts if people still don’t know where and how to access the data?
5.
Avoiding Data Traps: How to Prevent Organization Miscues
Data organization miscues occur when people, strategies, and capabilities lack alignment and focus. A miscue could be anything from an unmotivated employee wasting time and energy on something that leadership didn’t prioritize to management failing to enable employees with proper resources. Here are three common data traps to avoid during your data productization efforts:
- Leaders and directors need to understand and communicate the goals, the structure, and, most importantly, the intent to employees. The intent should also be aligned with how to measure success. Is success measured by cost savings? Speed to market? Attrition? Different metrics drive different behaviors depending on what employees are incentivized to achieve. If your focus is attrition, then user experience becomes the most important goal. If your focus is speed to market, the cost isn’t as compressed.
- Align employee skills with the needs of the organization. By choosing the right person, they can determine how to achieve the organizational goals by applying their skills to maximize value. If you need predictive analytics, consider a data scientist. If you need an interactive tool for consumers, consider a user researcher and designer. However, using a data scientist just to dashboard devalues their skills and will ultimately, demotivate the employee.
- Employ proper communication and training. Ensure that policies and best practices are shared and reiterated so that associates know where to go, when to perform certain actions, and how to find the right data. This will prevent silos from forming and maximize productization by enabling visibility and sharing of data products into untapped areas in the organization. If you don’t already have a data catalog and master data definitions, you need them. An employee who doesn’t know where to find data wastes time and value-add opportunities.
Examples of Successful Data Products and How They Reached More Customers
AIRBNB disrupted the hospitality market by renting rooms and homes to people who were traveling. The company’s existing data product includes where customers are staying and for how long. Airbnb used this data to enter a new market by adding a complementary product, Experiences, where customers can also purchase events, meals, and other activities in addition to their accommodations.3
LINKEDIN disrupted the recruiting industry in 2011 when it launched “Talent Pipeline,” now known as “Recruiter.” The company’s database included profiles of 100 million members at the time. By tapping into its existing data, LinkedIn was able to add a complementary service and extend its reach into an adjacent industry.6
FITBIT, a smartwatch and activity tracking company, collects and provides their customer’s data on steps, heart rate, sleep, distance traveled, calories burned, and more. The company realized its existing data is extremely valuable to other industries, such as insurance companies. Humana aims to distribute Fitbits to Medicare Advantage plan members to encourage healthy behavior and even alert users of potential health concerns. It’s a win across the board: Fitbit expanded the reach of its existing data; Humana potentially reduces health care costs; and consumers improve their health while potentially reducing their health care costs with more preventative measures.4
City planners need to understand how traffic flows and where people travel within a city to improve transportation. That’s why Uber launched a site called UBER Movement that includes a searchable database of historical trip data from every city in which it conducts business.5 Similarly, Uber entered the food delivery industry by applying its existing model and data capabilities to seamlessly add this new service.6
Final Thoughts
The ability to productize data starts with a strong data platform and governance framework. This model allows you to tap into new data products, markets, and industries by empowering associates to know where to find data, how to use it, and encouraging them to share a data product with new teams or external customers.
Success at face value includes sales, profit, customer satisfaction, return on investment, etc. Data productization goes far beyond the obvious. The real value lies in the ability to apply your data to new products, markets, or industries. The questions you should always ask yourself are “Who else would benefit from seeing this data?” and “What new markets can I enter with this data?”
Sources:
- HSG Analytics, 2020
- “The DGI Data Governance Framework”, prepared by Gwen Thomas, Data Governance Institute
- https://www.ideatovalue.com/inno/nickskillicorn/2019/07/ten-types-of-innovation-30-new-case-studies-for-2019/
- https://www.fiercehealthcare.com/payers/rise-2022-humana-aims-use-data-fitbits-help-some-medicare-advantage-members-predict-adverse
- https://www.investopedia.com/articles/investing/030916/how-uber-uses-its-data-bank.asp
- https://singularityhub.com/2015/10/26/12-industries-disrupted-by-tech-companies-expanding-into-new-markets/